Ensemble classification for identifying neighbourhood sources of fugitive dust and associations with observed PM10
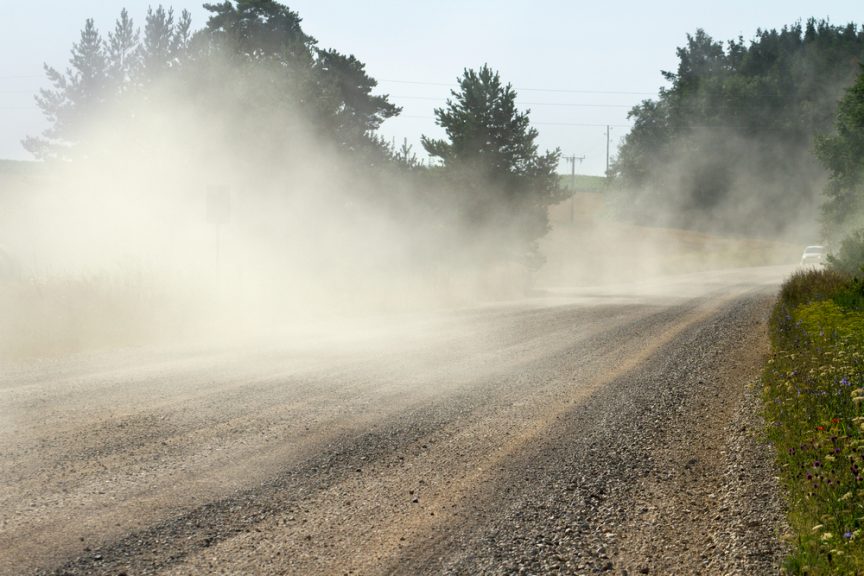
20 April 2017
English
uKESA Librarian, Sibusisiwe Makhanya
Journal article
Council for Scientific and Industrial Research
Africa
In urban areas the deterioration of air quality as a result of fugitive dust receives less attention than the more prominent traffic and industrial emissions. We assessed whether fugitive dust emission sources in the neighbourhood of an air quality monitor are predictors of ambient PM10 concentrations on days characterized by strong local winds. An ensemble maximum likelihood method is developed for land cover mapping in the vicinity of an air quality station using SPOT 6 multi-spectral images. The ensemble maximum likelihood classifier is developed through multiple training iterations for improved accuracy of the bare soil class. Five primary land cover classes are considered, namely built-up areas, vegetation, bare soil, water and ‘mixed bare soil’ which denotes areas where soil is mixed with either vegetation or synthetic materials.
Preliminary validation of the ensemble classifier for the bare soil class results in an accuracy range of 65–98%. Final validation of all classes results in an overall accuracy of 78%. Next, cluster analysis and a varying intercepts regression model are used to assess the statistical association between land cover, a fugitive dust emissions proxy and observed PM10. We found that land cover patterns in the neighbourhood of an air quality station are significant predictors of observed average PM10 concentrations on days when wind speeds are conducive for dust emissions. This study concludes that in the absence of an emissions inventory for the ambient particulate matter, PM10 emitted from dust reservoirs can be statistically accounted for by land cover characteristics. This supports the use of land cover data for improved prediction of PM10 at locations without air quality monitoring stations.
Comments